
- PDF TEXT EXTRACTOR PYTHON HOW TO
- PDF TEXT EXTRACTOR PYTHON PDF
- PDF TEXT EXTRACTOR PYTHON PROFESSIONAL
- PDF TEXT EXTRACTOR PYTHON SERIES
Most of the time invested in improving the models’ performances was dedicated to the enhancement of the hand-crafted features, not to reducing the models complexity or to fine-tuning. Each model is trained with hand-crafted features which describe textlines or textblocks themselves (unary features) or their relations with other elements (relative features). We implemented machine learning models in order to merge textlines into textblocks and classify them. Using feature engineering and machine learning to structure documents
PDF TEXT EXTRACTOR PYTHON PDF
We also know which sentences are titles, and how titles are hierarchized.īut for a computer a PDF file is simply a list of characters, which are not always ordered.
PDF TEXT EXTRACTOR PYTHON HOW TO
Understanding the problemīecause we learned how to read and write, we understand document layouts, from which letters form words, words form sentences, sentences form paragraphs and so on.
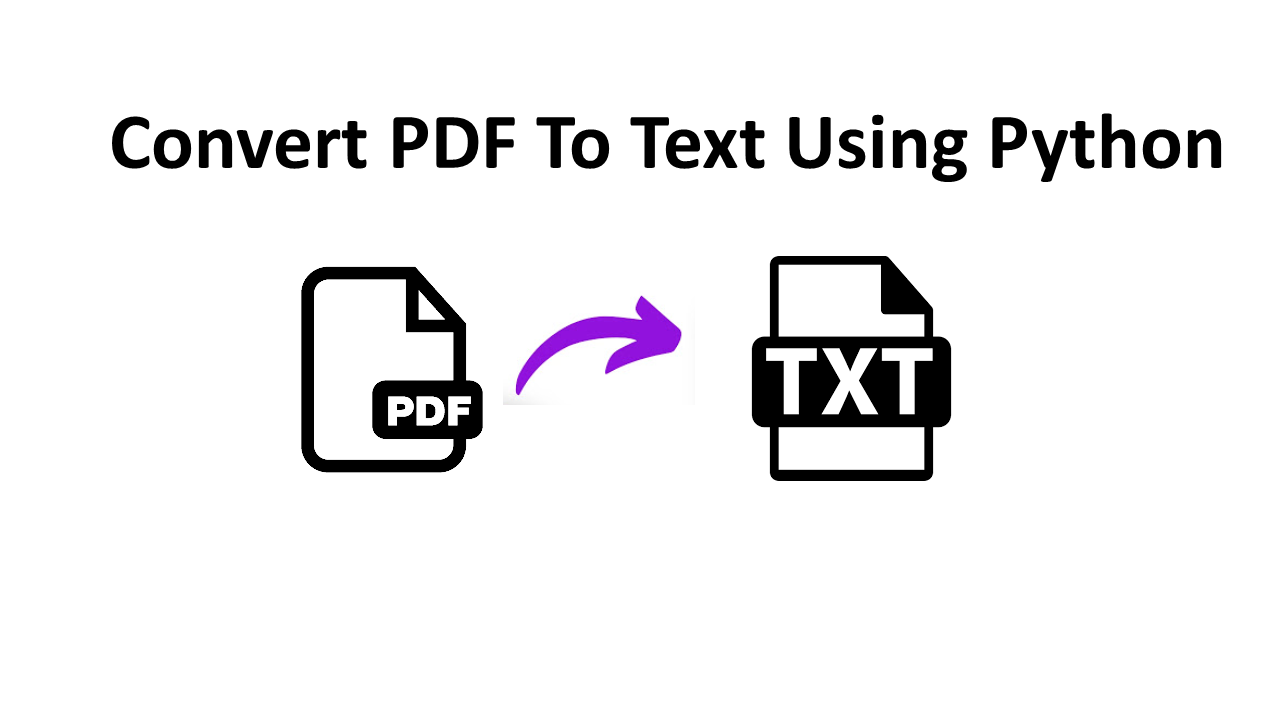
Within text strings, characters are shown using character codes (integers) that map to glyphs in the current font using an encoding. Unlike image PDF (scanned PDF) which require OCR, searchable PDF usually contains everything needed for parsing. PDFs also support arbitrary vector graphics as illustrations. Since the fonts are in vector format they are extremely compact and the size can be enlarged without losing sharpness. Searchable PDF are like a text files, they only store the needed characters of the fonts and the layout of the text on each page.
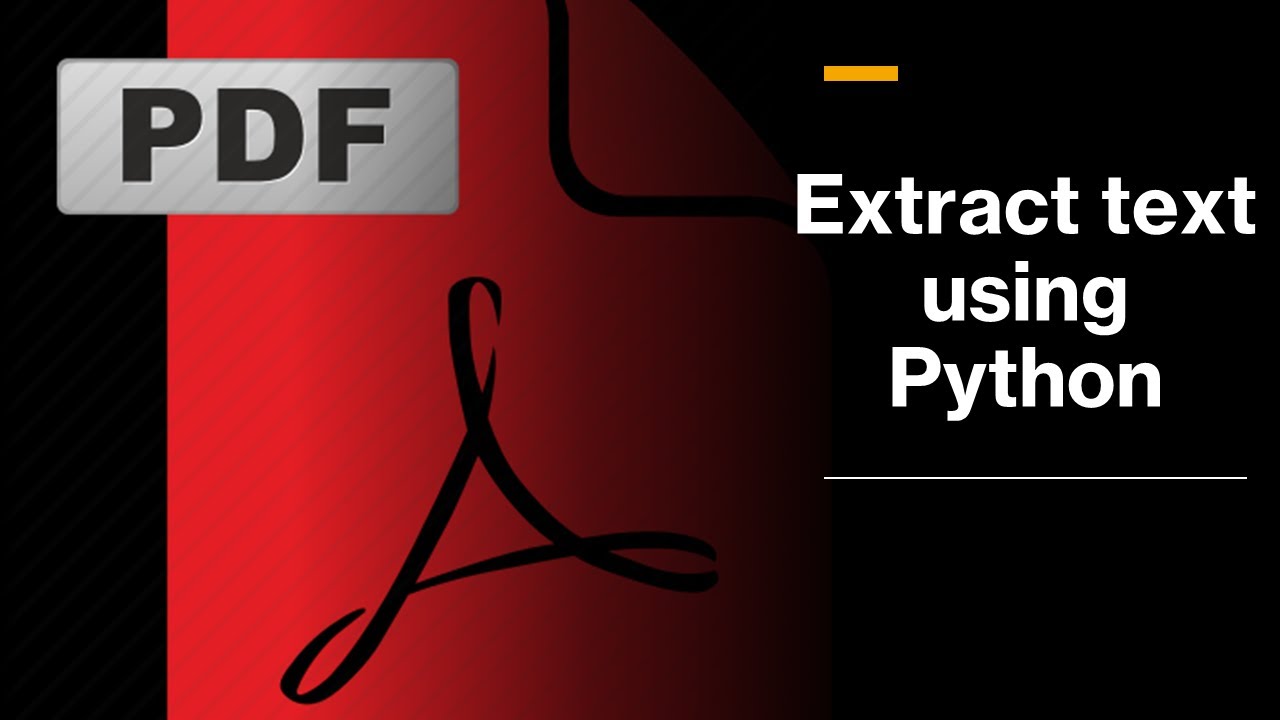
PDF TEXT EXTRACTOR PYTHON PROFESSIONAL
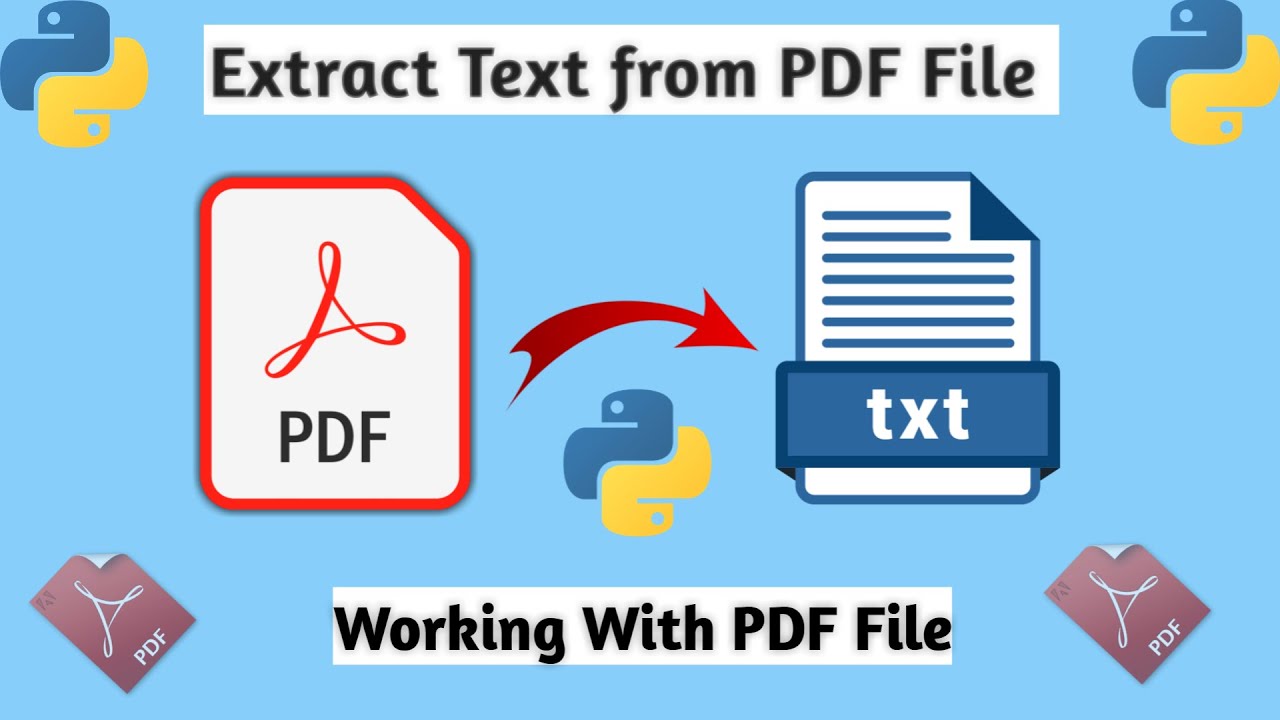
We built an end-to-end pipeline, which collects and transforms data into up-to-date medical knowledge. The third article details how NLP can be used to improve the accuracy of a medical search engine.The second article describes the Named-Entity Recognition (NER) and Named-Entity Linking (NEL) models that we built.The first article explains why it is necessary to train domain-specific word embedding and how Posos does it.
PDF TEXT EXTRACTOR PYTHON SERIES
If you are interested about our search engine, we published a series of three articles on how medical-specific NLP tools can be built and used.
